Quantitative Recognition of Pipeline Defects based on Neural Network and Data Fusion

摘 要
分析了管道缺陷的特征参数与漏磁信号的关系,研究显示管道缺陷的深度和长度分别与漏磁信号的幅值和宽度呈近似线性关系。将实际漏磁信号预处理以消除传感器提离值不同带来的影响,然后用已训练好的BP神经网络进行了管道缺陷的定量识别,识别结果的误差<10%,完全满足实际检测要求。分别用加权平均和自适应加权平均两种方法将轴向和径向漏磁信号进行信号级融合,融合后基于BP神经网络的缺陷定量识别的精度和可靠性得到了明显提高,其中加权平均法更优。








Abstract
Quantitative recognition of defect was the difficulty in pipeline magnetic flux leakage(MFL) inspection. The relationship between pipeline defects and MFL signals were studied. The study showed that the depth and length of the defects had an approximately linear relation to the amplitude and width of MFL signals respectively. The real MFL signals were preprocessed to eliminate the effects of sensor lift-off and then were recognized quantitatively by BP neural network already trained. The recognition result error was less than 10%, and practical inspection requirement was completely fulfilled. In order to get better recognition result, the axial and radial MFL signal were fused at signal level by weighted average and adaptive weighted average methods respectively. The accuracy and reliability of quantitative recognition based on BP neural network were improved remarkably after signal fusion. The results showed that the weighted average method was better than the adaptive weighted average method.
中图分类号 TG115.28
所属栏目 科研成果与学术交流
基金项目 国家自然科学基金资助项目(60327001)
收稿日期 2005/5/8
修改稿日期
网络出版日期

作者单位点击查看

备注杨理践(1957~),男,教授,博士生导师。
引用该论文: YANG Li-jian,MA Feng-ming,GAO Song-wei. Quantitative Recognition of Pipeline Defects based on Neural Network and Data Fusion[J]. Nondestructive Testing, 2006, 28(6): 281~284
杨理践,马凤铭,高松巍. 基于神经网络及数据融合的管道缺陷定量识别[J]. 无损检测, 2006, 28(6): 281~284

被引情况:

【1】杨 涛,王启江,高殿斌, "抽油管在线漏磁检测系统信号分析设计",无损检测 29, 447-449(2007)
【2】宋小春,黄松岭,康宜华,赵 伟, "漏磁无损检测中的缺陷信号定量解释方法",无损检测 29, 407-411(2007)
共有人对该论文发表了看法,其中:
人认为该论文很差
人认为该论文较差
人认为该论文一般
人认为该论文较好
人认为该论文很好
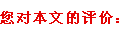





参考文献
【1】杨理践,王玉梅,冯海英.智能化管道漏磁检测装置的研究[J].无损检测,2002,24(3):100-102.
【2】余浩然,吴 斌,陈丽萍.漏磁通法油气管道在役检测技术[J].实用测试技术,1997,23(5):1-9.
【3】Hwang K, Mandayam S, Udpa SS, et al. Characterization of gas pipeline inspection signals using wavelet basis function neural networks[J]. NDT&E International,2000,33:531-545.
【4】Ramuhalli P, Afzal M, Hwang K-T, et al. A feedback neural network approach for electromagnetic NDE signal inversion[A]. Electromagnetic Nondestructive Evaluation(IV)-Studies in Applied Electromagnetics and Mechanics 17\[C\]. Udpa SS, Takagi T, Pavo J, et al, edited, The Netherlands: IOS Press,2000.1-8.
【5】杨 涛,王太勇,李 清,等.基于特征量和神经网络的钢管缺陷预测模型[J].钢铁,2004,39(9):50-53.
【2】余浩然,吴 斌,陈丽萍.漏磁通法油气管道在役检测技术[J].实用测试技术,1997,23(5):1-9.
【3】Hwang K, Mandayam S, Udpa SS, et al. Characterization of gas pipeline inspection signals using wavelet basis function neural networks[J]. NDT&E International,2000,33:531-545.
【4】Ramuhalli P, Afzal M, Hwang K-T, et al. A feedback neural network approach for electromagnetic NDE signal inversion[A]. Electromagnetic Nondestructive Evaluation(IV)-Studies in Applied Electromagnetics and Mechanics 17\[C\]. Udpa SS, Takagi T, Pavo J, et al, edited, The Netherlands: IOS Press,2000.1-8.
【5】杨 涛,王太勇,李 清,等.基于特征量和神经网络的钢管缺陷预测模型[J].钢铁,2004,39(9):50-53.
相关信息