Recognition of Defect in TOFD Image Based on Faster Region Convolutional Neural Networks

摘 要
受扫查图像效果和检测人员能力与主观因素的影响,检测人员在使用超声衍射时差法(TOFD)的D扫描图像判断焊缝缺陷类型时,存在可靠性低、争议大、速度慢的问题。为了提高缺陷类型识别的准确性及效率,分析了焊缝缺陷的TOFD-D扫描图像特征,构建了一种基于区域的卷积神经网络(Faster RCNN),对焊缝缺陷D扫描图像中的缺陷类型进行自动识别;在网络训练过程中,提出了采用样本扩展及建议框优化方法以提高网络训练的稳定性及效率。结果表明:缺陷的TOFD-D扫描图像的轮廓与缺陷几何形状密切相关,可用于判断缺陷类型;Faster RCNN网络虽然可能对界面波及噪声造成误判,但对缺陷类型的识别率可达到97%以上,可实现缺陷类型的自动识别,并具有高识别率、鲁棒性和抗干扰能力。








Abstract
Manual recognition of the type of weld defects through ultrasonic Time of Flight Diffraction (TOFD) image has disadvantages of low efficiency and reliability, due to the limitation of experience and knowledge of the tester. In order to improve the accuracy and efficiency of defect's identification, characteristics of weld defect of TOFD-D scan imagery were analyzed, and Faster Region based Convolutional Neural Networks(Faster RCNN) was proposed to auto-recognize the defect's type. Besides, the methods of proposed box optimization and sample expanding were also explored to improve training efficiency and stability. Finally, recognition effect and influences were analyzed. Research results show that D-scan image of the weld defect is closely related to the defect's geometry which can be used to distinguish the defect's type. Although the Faster RCNN network may misjudge noise to the interface, auto-recognition of the defect's type can be implemented through Faster RCNN with high recognition rate, robustness and anti-jamming ability, in which recognition accuracy of more than 97% is achieved.
中图分类号 TG156 TN06 TG115.28 DOI 10.11973/wsjc201907004
所属栏目 试验研究
基金项目 宁波市特种设备检验研究院院级项目(Y-2016-013)
收稿日期 2019/3/4
修改稿日期
网络出版日期

作者单位点击查看


备注黄焕东(1979-),硕士,高级工程师,主要从事承压类特种设备检验检测工作
引用该论文: HUANG Huandong,HU Lichen,LI Binbin,SHEN Chengye,WANG Hongyuan,CHEN Zhenhua. Recognition of Defect in TOFD Image Based on Faster Region Convolutional Neural Networks[J]. Nondestructive Testing, 2019, 41(7): 12~18
黄焕东,胡利晨,李斌彬,沈成业,王红源,陈振华. 基于区域的快速卷积神经网络的焊缝TOFD检测缺陷识别[J]. 无损检测, 2019, 41(7): 12~18
共有人对该论文发表了看法,其中:
人认为该论文很差
人认为该论文较差
人认为该论文一般
人认为该论文较好
人认为该论文很好
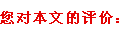





参考文献
【1】迟大钊, 刚铁, 袁媛, 等. 面状缺陷超声TOFD法信号和图像的特征与识别[J]. 焊接学报, 2005, 26(11):1-4.
【2】盛朝阳, 刚铁, 迟大钊, 等. 基于分水岭方法的超声TOFD检测图像分割[J]. 机械工程学报, 2011, 47(8):35-40.
【3】陈振华, 胡怀辉, 卢超, 等. 基于超声TOFD直通波及神经网络的近表面缺陷自动识别[J]. 无损检测, 2014, 36(3):14-17, 24.
【4】林乃昌, 杨晓翔, 唐旭晟, 等. 基于Gabor小波的TOFD图像缺陷识别研究[J]. 机电工程, 2013, 30(12):1450-1454.
【5】CRUZ F C, SIMAS FILHO E F, ALBUQUERQUE M C S, et al. Efficient feature selection for neural network based detection of flaws in steel welded joints using ultrasound testing[J]. Ultrasonics, 2017, 73:1-8.
【6】LIU J, XU G C, REN L, et al. Defect intelligent identification in resistance spot welding ultrasonic detection based on wavelet packet and neural network[J]. The International Journal of Advanced Manufacturing Technology, 2017, 90(9):2581-2588.
【7】孙志军, 薛磊, 许阳明, 等. 深度学习研究综述[J]. 计算机应用研究, 2012, 29(8):2806-2810.
【8】SCHMIDHUBER J. Deep learning in neural networks:An overview[J]. Neural Networks, 2015, 61:85-117.
【9】GUO Y M, LIU Y, OERLEMANS A, et al. Deep learning for visual understanding:a review[J]. Neurocomputing, 2016, 187:27-48.
【10】MENG M, CHUA Y J, WOUTERSON E, et al. Ultrasonic signal classification and imaging system for composite materials via deep convolutional neural networks[J]. Neurocomputing, 2017, 257:128-135.
【11】GIRSHICK R, DONAHUE J, DARRELL T, et al. Region-based convolutional networks for accurate object detection and segmentation[J]. IEEE Transactions on Pattern Analysis and Machine Intelligence, 2016, 38(1):142-158.
【12】RUTA A, LI Y M, LIU X H. Real-time traffic sign recognition from video by class-specific discriminative features[J].Pattern Recognition,2010,43(1):416-430.
【13】王万国, 田兵, 刘越, 等. 基于RCNN的无人机巡检图像电力小部件识别研究[J]. 地球信息科学学报, 2017, 19(2):256-263.
【14】ANTHIMOPOULOS M, CHRISTODOULIDIS S, EBNER L, et al. Lung pattern classification for interstitial lung diseases using a deep convolutional neural network[J]. IEEE Transactions on Medical Imaging, 2016, 35(5):1207-1216.
【2】盛朝阳, 刚铁, 迟大钊, 等. 基于分水岭方法的超声TOFD检测图像分割[J]. 机械工程学报, 2011, 47(8):35-40.
【3】陈振华, 胡怀辉, 卢超, 等. 基于超声TOFD直通波及神经网络的近表面缺陷自动识别[J]. 无损检测, 2014, 36(3):14-17, 24.
【4】林乃昌, 杨晓翔, 唐旭晟, 等. 基于Gabor小波的TOFD图像缺陷识别研究[J]. 机电工程, 2013, 30(12):1450-1454.
【5】CRUZ F C, SIMAS FILHO E F, ALBUQUERQUE M C S, et al. Efficient feature selection for neural network based detection of flaws in steel welded joints using ultrasound testing[J]. Ultrasonics, 2017, 73:1-8.
【6】LIU J, XU G C, REN L, et al. Defect intelligent identification in resistance spot welding ultrasonic detection based on wavelet packet and neural network[J]. The International Journal of Advanced Manufacturing Technology, 2017, 90(9):2581-2588.
【7】孙志军, 薛磊, 许阳明, 等. 深度学习研究综述[J]. 计算机应用研究, 2012, 29(8):2806-2810.
【8】SCHMIDHUBER J. Deep learning in neural networks:An overview[J]. Neural Networks, 2015, 61:85-117.
【9】GUO Y M, LIU Y, OERLEMANS A, et al. Deep learning for visual understanding:a review[J]. Neurocomputing, 2016, 187:27-48.
【10】MENG M, CHUA Y J, WOUTERSON E, et al. Ultrasonic signal classification and imaging system for composite materials via deep convolutional neural networks[J]. Neurocomputing, 2017, 257:128-135.
【11】GIRSHICK R, DONAHUE J, DARRELL T, et al. Region-based convolutional networks for accurate object detection and segmentation[J]. IEEE Transactions on Pattern Analysis and Machine Intelligence, 2016, 38(1):142-158.
【12】RUTA A, LI Y M, LIU X H. Real-time traffic sign recognition from video by class-specific discriminative features[J].Pattern Recognition,2010,43(1):416-430.
【13】王万国, 田兵, 刘越, 等. 基于RCNN的无人机巡检图像电力小部件识别研究[J]. 地球信息科学学报, 2017, 19(2):256-263.
【14】ANTHIMOPOULOS M, CHRISTODOULIDIS S, EBNER L, et al. Lung pattern classification for interstitial lung diseases using a deep convolutional neural network[J]. IEEE Transactions on Medical Imaging, 2016, 35(5):1207-1216.
相关信息