A method of ultrasonic signal feature extraction based on wavelet packet and principal component analysis

摘 要
为了有效识别不同类型的超声缺陷信号,提出了一种基于小波包分解和主成分分析(Principal Component Analysis,PCA)的信号特征提取方法。首先,提取缺陷信号小波包分解后的能量系数组成多维特征向量集;然后,使用PCA方法对多维特征向量进行降维得到融合特征量;最后,输入BP神经网络对不同类型的缺陷信号进行分类测试,并与未经PCA处理的特征量分类测试结果进行对比。试验结果证明,该特征量提取的方法能够有效地对缺陷进行分类,且测试速度明显得到提高。










Abstract
In order to effectively identify different types of ultrasonic defect signals, a method based on wavelet packet decomposition and principal component analysis was proposed to extract the characteristic quantities. Firstly, the multi-dimensional eigenvector set is composed of the energy coefficients of wavelet packet decomposition. Then the multidimensional feature vectors are reduced by PCA method to get the fusion feature quantity. Finally, BP neural network was used to classify and test different types of defect signals, and the obtained results were compared with the classification test results of characteristic quantities without PCA processing. The experimental results show that the feature extraction method can classify the defect types effectively, and the testing speed is obviously improved.
中图分类号 TP18 TG115.28 DOI 10.11973/wsjc201912009
所属栏目 试验研究
基金项目
收稿日期 2019/3/11
修改稿日期
网络出版日期

作者单位点击查看


备注肖力伟(1994-),硕士研究生,主要从事无损检测技术研究
引用该论文: XIAO Liwei. A method of ultrasonic signal feature extraction based on wavelet packet and principal component analysis[J]. Nondestructive Testing, 2019, 41(12): 41~44
肖力伟. 一种基于小波包和主成分分析的超声信号特征提取方法[J]. 无损检测, 2019, 41(12): 41~44
共有人对该论文发表了看法,其中:
人认为该论文很差
人认为该论文较差
人认为该论文一般
人认为该论文较好
人认为该论文很好
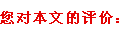





参考文献
【1】孙彬,王建华,赫东锋,等.基于激光测量的航发叶片表面几何缺陷识别技术[J].自动化学报,2018(12):1-6.
【2】李茂,杨录,张艳花.基于EMD及主成分分析的缺陷超声信号特征提取研究[J].中国测试,2018,44(2):118-121,133.
【3】戴波,赵晶,周炎.超声波管道内检测腐蚀缺陷分类识别研究[J].机床与液压,2008,36(7):194-198,240.
【4】郑善朴,陆铭慧,王俊东,等.多层结构中脱粘缺陷的超声检测方法[J].应用声学,2019,38(1):134-141.
【5】李娟,杨录,张艳花.基于EMD与欧氏距离的缺陷信号识别方法[J].山西电子技术,2011(2):35-36,41.
【6】杨琳瑜,于润桥,卢超,等.基于BP神经网络的复合材料超声波检测缺陷类型识别[J].无损检测,2007,29(8):450-452,460.
【7】GOODSON K E. Measurement of the thermal conductivityand heat capacity of freestanding shape memory thinfilms using the 3 method[J]. Journal of Heat Transfer,2008,130(10):2402-2409.
【8】罗来齐. 管道缺陷检测与识别方法研究与实现[D].镇江:江苏大学,2016.
【9】刘芳,高兴,周冰,等.基于PCA-ISVM的软件缺陷预测模型[J].计算机仿真,2014,31(3):397-401.
【10】唐东林,魏子兵,潘峰,等.基于PCA和SVM的管道腐蚀超声内检测[J].传感技术学报,2018,31(7):1040-1045.
【11】ZHANG H, LI M,FANG W,et al. A numerical study on the theoretical accuracy of film thermal conductivity using transient plane source method[J]. Applied Thermal Engineering,2014,72(1):62-69.
【12】KERR L, PAN Y, DINWIDDIE R, et al. Thermal conductivityof coated paper[J]. International Journal of Thermophysics,2009,30(2):572-579.
【2】李茂,杨录,张艳花.基于EMD及主成分分析的缺陷超声信号特征提取研究[J].中国测试,2018,44(2):118-121,133.
【3】戴波,赵晶,周炎.超声波管道内检测腐蚀缺陷分类识别研究[J].机床与液压,2008,36(7):194-198,240.
【4】郑善朴,陆铭慧,王俊东,等.多层结构中脱粘缺陷的超声检测方法[J].应用声学,2019,38(1):134-141.
【5】李娟,杨录,张艳花.基于EMD与欧氏距离的缺陷信号识别方法[J].山西电子技术,2011(2):35-36,41.
【6】杨琳瑜,于润桥,卢超,等.基于BP神经网络的复合材料超声波检测缺陷类型识别[J].无损检测,2007,29(8):450-452,460.
【7】GOODSON K E. Measurement of the thermal conductivityand heat capacity of freestanding shape memory thinfilms using the 3 method[J]. Journal of Heat Transfer,2008,130(10):2402-2409.
【8】罗来齐. 管道缺陷检测与识别方法研究与实现[D].镇江:江苏大学,2016.
【9】刘芳,高兴,周冰,等.基于PCA-ISVM的软件缺陷预测模型[J].计算机仿真,2014,31(3):397-401.
【10】唐东林,魏子兵,潘峰,等.基于PCA和SVM的管道腐蚀超声内检测[J].传感技术学报,2018,31(7):1040-1045.
【11】ZHANG H, LI M,FANG W,et al. A numerical study on the theoretical accuracy of film thermal conductivity using transient plane source method[J]. Applied Thermal Engineering,2014,72(1):62-69.
【12】KERR L, PAN Y, DINWIDDIE R, et al. Thermal conductivityof coated paper[J]. International Journal of Thermophysics,2009,30(2):572-579.
相关信息