Intelligent guided wave damage detection and assessment of stiffened plate

摘 要
建立了基于卷积神经网络算法的智能导波损伤检测方法,可实现加筋板中脱黏损伤的高效识别和精准定位。在数值模拟和试验研究T型筋加筋板中导波传播特性的基础上,通过单点激发多点接收的方法获取不同损伤样本的兰姆波响应,经预处理之后组成融合数据库。利用卷积神经网络(CNN)深度学习检测算法,抓取和学习融合数据库中与损伤相关的特征,并使用未经训练的数据测试网络性能。结果表明,以Adam为优化器的7层CNN对数据库中损伤样本的检测精度达99%;基于CNN的智能导波检测方法不仅能够识别加筋板中的脱黏损伤,而且能够准确定位。








Abstract
In this paper, an intelligent guided wave damage detection method based on convolutional neural network algorithm is established to realize efficient identification and precise positioning of debond damage in stiffened plates. Based on numerical simulation and experimental study on the propagation characteristics of guided wave in T-stiffened plate, the Lamb wave responses of different damaged samples are obtained by the method of single-point excitation and multi-point reception, and a fusion database is formed after preprocessing. The convolutional neural network (CNN) deep learning detection algorithm is used to extract and learn damage-related features in the fusion database, and the performance of the network is tested with untrained data. The results show that the 7-layer CNN with Adam as the optimizer can detect damage samples in the database with an accuracy of 99%. The CNN-based intelligent guided wave detection method for stiffened plates can not only identify debonding damage, but also accurately locate it.
中图分类号 O426.9 TG115.28 DOI 10.11973/wsjc202203003
所属栏目 试验与研究
基金项目 国家自然科学基金资助项目(62071205)
收稿日期 2021/6/3
修改稿日期
网络出版日期

作者单位点击查看

联系人作者许伯强(bqxu@ujs.edu.cn)

备注申庆(1995-),男,硕士研究生,主要研究方向为基于深度学习的超声导波损伤检测
引用该论文: SHEN Qing,XU Baiqiang,YUE Shengyao,XU Guidong,XU Chenguang,ZHANG Sai. Intelligent guided wave damage detection and assessment of stiffened plate[J]. Nondestructive Testing, 2022, 44(3): 12~17
申庆,许伯强,岳圣尧,徐桂东,徐晨光,张赛. 加筋板智能导波损伤识别与评估[J]. 无损检测, 2022, 44(3): 12~17
共有人对该论文发表了看法,其中:
人认为该论文很差
人认为该论文较差
人认为该论文一般
人认为该论文较好
人认为该论文很好
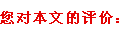





参考文献
【1】韩伟,冯侃,骆英.基于超声兰姆波的双曲线定位损伤成像方法[J].无损检测,2021,43(4):46-50.
【2】孙洪宇,彭丽莎,屈凯峰,等.机器学习在复合绝缘子缺陷超声检测中的应用与展望[J].无损检测,2021,43(5):58-63.
【3】刘鹏飞,刘南希,李祚华,等.钢管混凝土构件的介质多相性及导波检测[J].无损检测,2021,43(5):43-48.
【4】郑跃滨,武湛君,雷振坤,等.基于超声导波的航空航天结构损伤诊断成像技术研究进展[J].航空制造技术,2020,63(18):24-43.
【5】李一博,刘圆圆,芮小博.板状材料上加强筋的几何形状对兰姆波透射特性的影响[J].声学学报,2019,44(2):231-240.
【6】RICCI F,MONACO E,MAIO L,et al.Guided waves in a stiffened composite laminate with a delamination[J].Structural Health Monitoring,2016,15(3):351-358.
【7】李锐华,潘玲,严辰,等.基于导波的大电机定子绝缘损伤检测实验研究[J].高电压技术,2014,40(5):1539-1545.
【8】徐亮.基于卷积神经网络的疲劳裂纹诊断[D].南京:南京航空航天大学,2019.
【9】ZARGAR S A,YUAN F G.Impact diagnosis in stiffened structural panels using a deep learning approach[J].Structural Health Monitoring,2021,20(2):681-691.
【10】张茹,柴亚南,张阿盈,等.含预制脱黏的加筋壁板损伤扩展[J].机械强度,2020,42(3):716-722.
【2】孙洪宇,彭丽莎,屈凯峰,等.机器学习在复合绝缘子缺陷超声检测中的应用与展望[J].无损检测,2021,43(5):58-63.
【3】刘鹏飞,刘南希,李祚华,等.钢管混凝土构件的介质多相性及导波检测[J].无损检测,2021,43(5):43-48.
【4】郑跃滨,武湛君,雷振坤,等.基于超声导波的航空航天结构损伤诊断成像技术研究进展[J].航空制造技术,2020,63(18):24-43.
【5】李一博,刘圆圆,芮小博.板状材料上加强筋的几何形状对兰姆波透射特性的影响[J].声学学报,2019,44(2):231-240.
【6】RICCI F,MONACO E,MAIO L,et al.Guided waves in a stiffened composite laminate with a delamination[J].Structural Health Monitoring,2016,15(3):351-358.
【7】李锐华,潘玲,严辰,等.基于导波的大电机定子绝缘损伤检测实验研究[J].高电压技术,2014,40(5):1539-1545.
【8】徐亮.基于卷积神经网络的疲劳裂纹诊断[D].南京:南京航空航天大学,2019.
【9】ZARGAR S A,YUAN F G.Impact diagnosis in stiffened structural panels using a deep learning approach[J].Structural Health Monitoring,2021,20(2):681-691.
【10】张茹,柴亚南,张阿盈,等.含预制脱黏的加筋壁板损伤扩展[J].机械强度,2020,42(3):716-722.
相关信息