Fusion segmentation method for pavement crack detection

摘 要
为同时获取路面裂缝的位置信息与分布路径,以及形状延展和密度信息等,对目标检测算法与图像分割算法的融合进行了研究,在分析了目标检测算法与图像分割算法的网络结构与特征融合方式后提出了一种基于YOLO V5与PSPnet的PSP-YOLO裂缝检测分割算法。同时针对裂缝图像采集困难、样本不足的问题提出一种基于GAN网络的数据扩增网络,生成以假乱真的裂缝图像对裂缝样本进行扩增。试验结果表明,PSP-YOLO检测分割算法能够同时获取裂缝的位置与形状延展等信息,在该数据集下,其路面裂缝检测的平均精度为93.18%,分割模块的平均交并比为74.68%。在相同的试验条件下,所提方法比原YOLO V5的平均精度提高了2.69%,分割模块的平均交并比比原PSPnet的提高了1.54%。










Abstract
In order to obtain the location information, distribution path, shape extension and density information of pavement cracks at the same time, the fusion of target detection algorithm and image segmentation algorithm is studied. After analyzing the network structure and feature fusion mode of target detection algorithm and image segmentation algorithm, a PSP-YOLO crack detection and segmentation algorithm based on YOLO V5 and PSPnet is proposed. At the same time, a data augmentation network based on GAN network is proposed to generate false fracture images to augment fracture samples. The experimental results show that the PSP-YOLO detection and segmentation algorithm can obtain the information of crack location and shape extension at the same time. The average accuracy of pavement crack detection under this data set is 93. 18%, and the average intersection over union of segmentation module is 74. 68%. Under the same experimental conditions, the average accuracy of the segmentation module is 2. 69% higher than that of the original YOLO V5, and the average intersection over union of the segmentation module is 1. 54% higher than that of the original PSP-net.
中图分类号 TG115.28 TP391.41 DOI 10.11973/wsjc202301001
所属栏目 试验研究
基金项目 国家重点研发计划(2019YFB1310000)
收稿日期 2022/6/23
修改稿日期
网络出版日期

作者单位点击查看


备注仝泽兴(1996-),男,硕士研究生,研究方向为机器视觉
引用该论文: TONG Zexing,LEI Bin,JIANG Lin,WANG Nianxian. Fusion segmentation method for pavement crack detection[J]. Nondestructive Testing, 2023, 45(1): 1~7
仝泽兴,雷斌,蒋林,王念先. 路面裂缝检测融合分割方法[J]. 无损检测, 2023, 45(1): 1~7
共有人对该论文发表了看法,其中:
人认为该论文很差
人认为该论文较差
人认为该论文一般
人认为该论文较好
人认为该论文很好
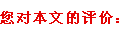





参考文献
【1】陈克鸿,陈冠雄.我国公路养护市场化的问题与发展建议[J].中国公路,2019(13):46-49.
【2】周基,蔡强,田琼.70年中国公路路基路面病害研究现状与发展趋势——基于CNKI 1949-2019年文献的知识图谱分析[J].中外公路,2020,40(3):60-66.
【3】李良福,马卫飞,李丽,等.基于深度学习的桥梁裂缝检测算法研究[J].自动化学报,2019,45(9):1727-1742.
【4】毛莺池,唐江红,王静,等.基于Faster R-CNN的多任务增强裂缝图像检测方法[J].智能系统学报,2021,16(2):286-293.
【5】REN S Q,HE K M,GIRSHICK R,et al.Faster R-CNN:towards real-time object detection with region proposal networks[J].IEEE Transactions on Pattern Analysis and Machine Intelligence,2017,39(6):1137-1149.
【6】李想,熊进刚.基于深度学习SSD目标检测算法的混凝土结构裂缝识别[J].南昌大学学报(工科版),2021,43(1):43-51.
【7】徐欢,李振璧,姜媛媛,等.基于OpenCV和改进Canny算子的路面裂缝检测[J].计算机工程与设计,2014,35(12):4254-4258.
【8】JI A K,XUE X L,WANG Y N,et al.An integrated approach to automatic pixel-level crack detection and quantification of asphalt pavement[J].Automation in Construction,2020,114:103176.
【9】高强,姜忠昊.基于GAN等效模型的小样本库扩增研究[J].电测与仪表,2019,56(6):76-81.
【10】WU S T,ZHONG S H,LIU Y.Deep residual learning for image steganalysis[J].Multimedia Tools and Applications,2018,77(9):10437-10453.
【2】周基,蔡强,田琼.70年中国公路路基路面病害研究现状与发展趋势——基于CNKI 1949-2019年文献的知识图谱分析[J].中外公路,2020,40(3):60-66.
【3】李良福,马卫飞,李丽,等.基于深度学习的桥梁裂缝检测算法研究[J].自动化学报,2019,45(9):1727-1742.
【4】毛莺池,唐江红,王静,等.基于Faster R-CNN的多任务增强裂缝图像检测方法[J].智能系统学报,2021,16(2):286-293.
【5】REN S Q,HE K M,GIRSHICK R,et al.Faster R-CNN:towards real-time object detection with region proposal networks[J].IEEE Transactions on Pattern Analysis and Machine Intelligence,2017,39(6):1137-1149.
【6】李想,熊进刚.基于深度学习SSD目标检测算法的混凝土结构裂缝识别[J].南昌大学学报(工科版),2021,43(1):43-51.
【7】徐欢,李振璧,姜媛媛,等.基于OpenCV和改进Canny算子的路面裂缝检测[J].计算机工程与设计,2014,35(12):4254-4258.
【8】JI A K,XUE X L,WANG Y N,et al.An integrated approach to automatic pixel-level crack detection and quantification of asphalt pavement[J].Automation in Construction,2020,114:103176.
【9】高强,姜忠昊.基于GAN等效模型的小样本库扩增研究[J].电测与仪表,2019,56(6):76-81.
【10】WU S T,ZHONG S H,LIU Y.Deep residual learning for image steganalysis[J].Multimedia Tools and Applications,2018,77(9):10437-10453.
相关信息