Infrared image identification method of building facade leakage based on YOLO V5 algorithm

摘 要
采用深度学习技术中的YOLO V5目标识别算法对红外成像仪中采集到的渗漏区域红外图像进行识别。对于红外渗漏目标而言,不同背景条件、不同时间的红外渗漏目标样本量较少且难以采集,给深度学习模型的训练造成了很大的困难。深度学习需要较多的检测目标数据量进行训练,为了减少对真实渗漏红外图像数量的需求,结合仿真渗漏红外图像与真实渗漏红外图像来制作数据集,作为深度学习的样本进行训练。试验结果表明,所提出的数据集制作与识别方法,对建筑外立面红外图像中渗漏区域的识别准确率达87.6%。








Abstract
In this paper, the YOLO V5 target recognition algorithm of the depth learning technology was used to identify the leakage region of the infrared imager from collected infrared images. For infrared leaky targets, the sample size of infrared leaky targets with different background conditions and different time was small and difficult to collect, which made the training of deep learning model very difficult. In order to reduce the need of real infrared leakage image, this paper combined simulated infrared leakage image with real infrared leakage image to make data set, train these data set as a sample for deep learning. The experimental results showed that the accuracy of the proposed method was 87. 6% for identifying the leakage area in the infrared image of the building facade.
中图分类号 TU761.11 TP39.141 TG115.28 DOI 10.11973/wsjc202310011
所属栏目 试验研究
基金项目 中央级公益性科研院所基本科研业务费专项资金(Y322008);国家重点研发计划(2022YFC3005502);国家自然科学基金(51979174);国家自然科学基金联合基金项目(U2040221)
收稿日期 2023/4/24
修改稿日期
网络出版日期

作者单位点击查看


备注吴玉龙(1988-),男,硕士,高级工程师,主要从事质量检测的相关工作
引用该论文: WU Yulong,WANG Jiahao,DING Yong. Infrared image identification method of building facade leakage based on YOLO V5 algorithm[J]. Nondestructive Testing, 2023, 45(10): 54~58
吴玉龙,王嘉浩,丁勇. 基于YOLO V5算法的建筑外立面渗漏红外图像识别方法[J]. 无损检测, 2023, 45(10): 54~58
共有人对该论文发表了看法,其中:
人认为该论文很差
人认为该论文较差
人认为该论文一般
人认为该论文较好
人认为该论文很好
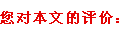





参考文献
【1】ZHONG,WANG Q M, LIN C,et al. Breaches of embankment and landslide dams-state of the art review[J]. Earth-Science Reviews: The International Geological Journal Bridging the Gap between Research Articles and Textbooks, 2021, 216(1):1-9.
【2】李宏恩,马桂珍,王芳,等.2000—2018年中国水库溃坝规律分析与对策[J].水利水运工程学报,2021(5):101-111.
【3】CHEN S S,ZHONG Q M,SHEN G Z.Numerical modeling of earthen dam breach due to piping failure[J].Water Science and Engineering,2019,12(3):169-178.
【4】许献磊,孙帅航,孙明浩,等.GPR技术在南水北调工程坝体渗漏探测中的应用[J].长江科学院院报,2020,37(10):59-63.
【5】朱德兵.土坝体隐患弹性波探测中的横波优势[J].地球物理学进展,2008,23(2):612-616.
【6】甘孝清,肖庆,宁晶.土石坝渗流热监测理论研究进展[J].长江科学院院报,2014,31(7):119-124.
【7】HIEN P D,VAN KHOI L.Application of isotope tracer techniques for assessing the seepage of the hydropower dam at Tri An,South Vietnam[J].Journal of Radioanalytical and Nuclear Chemistry,1996,206(2):295-303.
【8】李文忠,肖国强,孙卫民,等.长江堤防土电阻率测试及其与含水率和密实度的相关性研究[J].长江科学院院报,2019,36(10):131-134.
【9】龚起民.车载式隧道表面图像采集与病害检测技术[D].北京:北京交通大学,2020.
【10】杨玥旻,闫维新.基于图像处理的爬壁机器人焊缝识别与跟踪[J].机械与电子,2021,39(3):65-68,74.
【11】孙保燕,陈文.三维激光扫描和红外检测融合技术的工程应用[J].长江科学院院报,2020,37(2):170-173,178.
【12】余阳,赵海龙,韩来君.基于红外热成像的山火识别技术研究[J].现代电子技术,2017,40(24):77-79,84.
【13】KYLILI A,FOKAIDES P A,CHRISTOU P,et al.Infrared thermography (IRT) applications for building diagnostics:a review[J].Applied Energy,2014,134:531-549.
【14】BALARAS C A,ARGIRIOU A A.Infrared thermography for building diagnostics[J].Energy and Buildings,2002,34(2):171-183.
【15】JADIN M S,TAIB S.Recent progress in diagnosing the reliability of electrical equipment by using infrared thermography[J].Infrared Physics & Technology,2012,55(4):236-245.
【2】李宏恩,马桂珍,王芳,等.2000—2018年中国水库溃坝规律分析与对策[J].水利水运工程学报,2021(5):101-111.
【3】CHEN S S,ZHONG Q M,SHEN G Z.Numerical modeling of earthen dam breach due to piping failure[J].Water Science and Engineering,2019,12(3):169-178.
【4】许献磊,孙帅航,孙明浩,等.GPR技术在南水北调工程坝体渗漏探测中的应用[J].长江科学院院报,2020,37(10):59-63.
【5】朱德兵.土坝体隐患弹性波探测中的横波优势[J].地球物理学进展,2008,23(2):612-616.
【6】甘孝清,肖庆,宁晶.土石坝渗流热监测理论研究进展[J].长江科学院院报,2014,31(7):119-124.
【7】HIEN P D,VAN KHOI L.Application of isotope tracer techniques for assessing the seepage of the hydropower dam at Tri An,South Vietnam[J].Journal of Radioanalytical and Nuclear Chemistry,1996,206(2):295-303.
【8】李文忠,肖国强,孙卫民,等.长江堤防土电阻率测试及其与含水率和密实度的相关性研究[J].长江科学院院报,2019,36(10):131-134.
【9】龚起民.车载式隧道表面图像采集与病害检测技术[D].北京:北京交通大学,2020.
【10】杨玥旻,闫维新.基于图像处理的爬壁机器人焊缝识别与跟踪[J].机械与电子,2021,39(3):65-68,74.
【11】孙保燕,陈文.三维激光扫描和红外检测融合技术的工程应用[J].长江科学院院报,2020,37(2):170-173,178.
【12】余阳,赵海龙,韩来君.基于红外热成像的山火识别技术研究[J].现代电子技术,2017,40(24):77-79,84.
【13】KYLILI A,FOKAIDES P A,CHRISTOU P,et al.Infrared thermography (IRT) applications for building diagnostics:a review[J].Applied Energy,2014,134:531-549.
【14】BALARAS C A,ARGIRIOU A A.Infrared thermography for building diagnostics[J].Energy and Buildings,2002,34(2):171-183.
【15】JADIN M S,TAIB S.Recent progress in diagnosing the reliability of electrical equipment by using infrared thermography[J].Infrared Physics & Technology,2012,55(4):236-245.
相关信息