Stratified Analysis of the Magnetic Barkhausen Noise Signal Based on Wavelet Decomposition and Back Propagation Neural Network

摘 要
因小波变换具有多尺度分析的特点,在时频两域都有表征信号局部特征的能力<参考文献原文>,因此采用小波分解方法研究了不同时频成分的磁巴克豪森(MBN)信号随温度和应力变化的灵敏度问题。采用db5小波对MBN信号进行6层小波分解,提取各层分解系数的均值和均方根,并讨论分析了各特征值随所加应力以及温度变化的相对变化关系。研究表明,在试样的弹性范围内,低频系数和各层高频系数的均值和均方根都随压应力的增加而减小;各层高频系数的均值和均方根随温度的升高而降低,低频系数的均值和均方根随温度的升高而升高。最后将温度、原始MBN信号以及各分解系数的均值和均方根作为神经网络的输入,压应力作为其输出建立神经网络模型,结果表明该神经网络模型与之前没有用小波分解时的神经网络模型相比,检测应力的准确性更高。






Abstract
Because the wavelet transform has the characteristic of multi-scale analysis and it can characterize signals local feature <参考文献原文>, this article uses the wavelet decomposition method to study the sensitivity of different time-frequency components of the Magnetic Barkhausen Noise signal with changes in temperature and stress. After using the db5 wavelet with six layers to decompose the MBN signal, we extract the mean and RMS value of each layer decomposition coefficients and discuss the relationship between the relative change of the features and applied temperature and stress. It is found that within the elastic range of the sample, the mean and RMS value of high-frequency coefficients of each layer and low-frequency coefficients both decrease with increasing compressive stress. The mean and RMS value of high-frequency coefficients of each layer decrease, whereas the values of the low-frequency coefficients increase with increasing temperature respectively. This article takes temperature, the mean and RMS value of the original MBN signal and the decomposition coefficients as the input of the neural network and takes the stress as the output of the neural network to build the neural network model. It is shown that using this neural network model to detect stress has higher accuracy than the former BP neural network model in which the wavelet decomposition is not used.
中图分类号 TG115.28
所属栏目 2012远东无损检测新技术论坛论文精选
基金项目 国家自然科学基金资助项目(50907032);江苏省科技厅资助项目(BE2009162,BZ2009051);博士点基金资助项目(20093218120019)
收稿日期 2012/5/22
修改稿日期
网络出版日期

作者单位点击查看

备注姬小丽(1986-),女,硕士研究生,研究方向;无损检测。
引用该论文: JI Xiao-Li,WANG Ping,TIAN Gui-Yun,ZHU Lei. Stratified Analysis of the Magnetic Barkhausen Noise Signal Based on Wavelet Decomposition and Back Propagation Neural Network[J]. Nondestructive Testing, 2012, 34(11): 5~9
姬小丽,王平,田贵云,朱磊. 基于小波分解和BP神经网络的磁巴克豪森噪声信号分层分析研究[J]. 无损检测, 2012, 34(11): 5~9
共有人对该论文发表了看法,其中:
人认为该论文很差
人认为该论文较差
人认为该论文一般
人认为该论文较好
人认为该论文很好
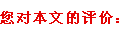





参考文献
【1】刑素霞.多光谱图像融合中小波分解层数研究[J].微电子学与计算机,2011,28(1):176-179.
【2】王骁,刘辉,祁欣,等.巴克豪森噪讯无缝线路应力检测仪的研制及应用[J].北京化工大学学报,2010,37(3):123-126.
【3】Barkhausen H. Two phenomena revealed with the help of new amplifiers[J]. Phys,1919,Z29:401.
【4】杨雅荣.基于巴克豪森效应的钢轨表面应力研究[C].2009远东无损检测论坛.苏州:2009.
【5】彭玉华.小波变换与工程应用[M].北京:科学出版社,2005.52-54
【6】朱寿高.基于巴克豪森噪声应力检测系统的研究[D].南京:南京航空航天大学,2009.
【7】朱秋君.基于BP神经网络的巴克豪森铁轨温度应力检测系统[C].2011远东无损检测论坛.杭州:2011.
【2】王骁,刘辉,祁欣,等.巴克豪森噪讯无缝线路应力检测仪的研制及应用[J].北京化工大学学报,2010,37(3):123-126.
【3】Barkhausen H. Two phenomena revealed with the help of new amplifiers[J]. Phys,1919,Z29:401.
【4】杨雅荣.基于巴克豪森效应的钢轨表面应力研究[C].2009远东无损检测论坛.苏州:2009.
【5】彭玉华.小波变换与工程应用[M].北京:科学出版社,2005.52-54
【6】朱寿高.基于巴克豪森噪声应力检测系统的研究[D].南京:南京航空航天大学,2009.
【7】朱秋君.基于BP神经网络的巴克豪森铁轨温度应力检测系统[C].2011远东无损检测论坛.杭州:2011.
相关信息