Optimization of Injection Process Parameters for Composite Parts by BP Neural Network and Genetic Algorithm

摘 要
以Moldflow软件模拟得到的不同工艺参数下飞机机头雷达罩模型的翘曲变形量为训练样本,在雷达罩模型成型工艺参数与其翘曲变形量间建立反向传播(Back Propagation,BP)神经网络模型,然后采用遗传算法对工艺参数进行优化,得到使雷达罩模型翘曲变形量最小的工艺参数并进行试验验证。结果表明:在相同工艺参数下由BP神经网络得到的雷达罩模型翘曲变形量与采用Moldflow软件模拟得到的翘曲变形量相近,相对误差小于4%,证明了BP神经网络的可靠性;模拟得到雷达罩模型的最优成型工艺参数为注塑温度295℃、模具温度80℃、注塑时间0.75 s、保压时间8 s、保压压力125 MPa,此时翘曲变形量最小,为0.121 3 mm;在最优成型工艺参数下进行注塑成型后得到的雷达罩模型最大翘曲变形量为0.126 0 mm,试验结果与预测结果间的相对误差小于3.7%,验证了BP神经网络与遗传算法相结合方法的准确性。








Abstract
Taking warpage deformation of the aircraft nose radome under different process parameters obtained by Moldflow software as training samples, a back propagation (BP) neural network model was established between the process parameters of the radome model and its warpage deformation values. Then genetic algorithm was used to optimize the process parameters, and the process parameters of the radome model with the smallest warpage deformation value was obtained. The results show that with the same process parameters, the warpage deformation value of the radome model obtained by BP neural network was similar to that simulated by Moldflow software, and the relative error was less than 4%, which proved the reliability of BP neural network. The simulated optimal molding process parameters of the radome model were injection temperature of 295 ℃, mold temperature of 80 ℃, injection time of 0.75 s, pressure holding time of 8 s, and pressure holding pressure of 125 MPa; the warpage deformation value was the smallest of 0.121 3 mm. The maximum warpage deformation of the radome model was 0.126 0 mm after injection molding with the optimal molding process parameters, and the ralative error between the experimental result and the predicted result was less than 3.7%, which verified the accuracy of the method of combining BP neural network and genetic algorithm.
中图分类号 TB332 DOI 10.11973/jxgccl202107012
所属栏目 物理模拟与数值模拟
基金项目 陕西省自然科学基础研究计划项目(2019JM-435)
收稿日期 2020/7/3
修改稿日期 2021/5/17
网络出版日期

作者单位点击查看

备注王博(1983-),男,陕西咸阳人,讲师,硕士
引用该论文: WANG Bo,ZHAO Dongping,LI Feng,ZHAO Shimin,WANG Yizhuo. Optimization of Injection Process Parameters for Composite Parts by BP Neural Network and Genetic Algorithm[J]. Materials for mechancial engineering, 2021, 45(7): 63~68
王博,赵东平,李锋,赵世民,王艺卓. 基于反向传播神经网络与遗传算法优化复合材料零件注塑成型工艺参数[J]. 机械工程材料, 2021, 45(7): 63~68
共有人对该论文发表了看法,其中:
人认为该论文很差
人认为该论文较差
人认为该论文一般
人认为该论文较好
人认为该论文很好
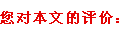





参考文献
【1】廖正品.我国塑料制品行业现状及发展热点分析[J].塑料工业,2013,41(增刊1):6-15. LIAO Z P. China's plastic products industry status and development hot spot analysis[J]. China Plastics Industry, 2013, 41(S1):22-30.
【2】丁永峰,龙婵娟.PC+ABS工程塑料合金薄壁制件注塑工艺参数的优化[J].机械工程材料,2019,43(11):12-15. DING Y F, LONG C J. Optimization of injection process parameters of PC+ABS engineering plastic alloy thin-walled parts[J]. Materials for Mechanical Engineering, 2019,43(11):12-15.
【3】李涛,严波,彭雄奇,等.玻纤增强注塑件的均匀化弹性力学参数研究[J].复合材料学报,2015,32(4):1153-1158. LI T, YAN B, PENG X Q, et al. Elastic properties of glass fiber reinforced injection moldings based on homogenization method[J]. Acta Materiae Compositae Sinica, 2015, 32(4):1153-1158.
【4】张友根.碳纤维复合材料推进汽车科学发展的分析研究[J]. 现代塑料加工应用,2015,27(6):60-63. ZHANG Y G. Analysis and research of carbon fiber composite materials for scientific development of automobile[J]. Modern Plastics Processing and Applications, 2015,27(6):60-63.
【5】王冠,王旭林,寇琳媛,等.基于多因子交互作用的PP/EPDM复合材料注射成型工艺优化[J].机械工程材料,2018, 42(3):87-94. WANG G,WANG X L,KOU L Y,et al.Optimization of PP/EPDM composite injection molding process based on multi-factor interaction[J].Materials for Mechanical Engineering,2018, 42(3):87-94.
【6】PATCHARAPHUN S,MENNIG G.Simulation and experimental investigations of material distribution in the sandwich injection molding process[J].Polymer-Plastics Technology and Engineering,2006,45(6):759-768.
【7】LOTTI C, UEKI M M, BRETAS R E S. Prediction of the shrinkage of injection molded iPP plaques using artificial neural networks[J]. Journal of Injection Molding Technology, 2002, 6(3):157-176.
【8】廖秋慧,刘淑梅.注塑成型保压参数对塑件翘曲变形的影响[J].塑料科技,2009, 37(10):67-69. LIAO Q H,LIU S M.Effect of packing parameters on warpage of moulded part during injection moulding[J].Plastics Science and Technology,2009, 37(10):67-69.
【9】申长雨,王利霞,张勤星.神经网络与混合遗传算法结合的注塑成型工艺优化[J]. 高分子材料科学与工程,2005,21(5):23-27. SHEN C Y, WANG L X, ZHANG Q X. Process optimization of injection molding by the combining ANN/HGA method[J]. Ploymer Materials Science and Engineering, 2005, 21(5):23-27.
【10】YIN F,MAO H J,HUA L.A hybrid of back propagation neural network and genetic algorithm for optimization of injection molding process parameters[J].Materials and Design,2011,32(6):3457-3464.
【11】王博,蔡安江,华荣佩,等.基于神经网络与正交试验的塑件翘曲变形优化[J].塑料,2019, 48(6):80-83. WANG B,CAI A J,HUA R P,et al.Optimization of the warpage for plastic parts based on neural network and orthogonal experiment[J].Plastics,2019, 48(6):80-83.
【12】时慧焯.基于人工神经网络的注塑成型翘曲优化方法[D]. 大连:大连理工大学,2012. SHI H Z. Warpage optimization methods based on artificial neural network in injection molding[D]. Dalian:Dalian University of Technology,2012.
【13】MIRIGUL A. Reducing shrinkage in injection moldings via the taguchi, anova and neural network methods[J]. Materials and Design, 2010, 31(1):599-604.
【14】郭广思.注塑成型技术[M]. 2版.北京:机械工业出版社,2009. GUO G S. Injection molding technology[M]. 2nd ed. Beijing:China Machine Press,2009.
【15】王博.基于CAE的电池盖注塑模具设计及成型工艺优化[J]. 中国塑料,2015, 29(8):106-110. WANG B. Injection mold design of battery cover and optimization of forming process based on CAE[J]. Chnia Plastics, 2015, 29(8):106-110.
【16】刘文卿.实验设计[M].北京:清华大学出版社,2005. LIU W Q.Design of experiments[M].Beijing:Tsinghua University Press,2005.
【17】ERFAN O, BEHZAD S, SEYED M, et al. Warpage and shrinkage optimization of injection-molded plastic spoon parts for biodegradable polymers using Taguchi,ANOVA and artificial neural network methods[J].Journal of Materials Science & Technology,2016, 32(8):710-720.
【2】丁永峰,龙婵娟.PC+ABS工程塑料合金薄壁制件注塑工艺参数的优化[J].机械工程材料,2019,43(11):12-15. DING Y F, LONG C J. Optimization of injection process parameters of PC+ABS engineering plastic alloy thin-walled parts[J]. Materials for Mechanical Engineering, 2019,43(11):12-15.
【3】李涛,严波,彭雄奇,等.玻纤增强注塑件的均匀化弹性力学参数研究[J].复合材料学报,2015,32(4):1153-1158. LI T, YAN B, PENG X Q, et al. Elastic properties of glass fiber reinforced injection moldings based on homogenization method[J]. Acta Materiae Compositae Sinica, 2015, 32(4):1153-1158.
【4】张友根.碳纤维复合材料推进汽车科学发展的分析研究[J]. 现代塑料加工应用,2015,27(6):60-63. ZHANG Y G. Analysis and research of carbon fiber composite materials for scientific development of automobile[J]. Modern Plastics Processing and Applications, 2015,27(6):60-63.
【5】王冠,王旭林,寇琳媛,等.基于多因子交互作用的PP/EPDM复合材料注射成型工艺优化[J].机械工程材料,2018, 42(3):87-94. WANG G,WANG X L,KOU L Y,et al.Optimization of PP/EPDM composite injection molding process based on multi-factor interaction[J].Materials for Mechanical Engineering,2018, 42(3):87-94.
【6】PATCHARAPHUN S,MENNIG G.Simulation and experimental investigations of material distribution in the sandwich injection molding process[J].Polymer-Plastics Technology and Engineering,2006,45(6):759-768.
【7】LOTTI C, UEKI M M, BRETAS R E S. Prediction of the shrinkage of injection molded iPP plaques using artificial neural networks[J]. Journal of Injection Molding Technology, 2002, 6(3):157-176.
【8】廖秋慧,刘淑梅.注塑成型保压参数对塑件翘曲变形的影响[J].塑料科技,2009, 37(10):67-69. LIAO Q H,LIU S M.Effect of packing parameters on warpage of moulded part during injection moulding[J].Plastics Science and Technology,2009, 37(10):67-69.
【9】申长雨,王利霞,张勤星.神经网络与混合遗传算法结合的注塑成型工艺优化[J]. 高分子材料科学与工程,2005,21(5):23-27. SHEN C Y, WANG L X, ZHANG Q X. Process optimization of injection molding by the combining ANN/HGA method[J]. Ploymer Materials Science and Engineering, 2005, 21(5):23-27.
【10】YIN F,MAO H J,HUA L.A hybrid of back propagation neural network and genetic algorithm for optimization of injection molding process parameters[J].Materials and Design,2011,32(6):3457-3464.
【11】王博,蔡安江,华荣佩,等.基于神经网络与正交试验的塑件翘曲变形优化[J].塑料,2019, 48(6):80-83. WANG B,CAI A J,HUA R P,et al.Optimization of the warpage for plastic parts based on neural network and orthogonal experiment[J].Plastics,2019, 48(6):80-83.
【12】时慧焯.基于人工神经网络的注塑成型翘曲优化方法[D]. 大连:大连理工大学,2012. SHI H Z. Warpage optimization methods based on artificial neural network in injection molding[D]. Dalian:Dalian University of Technology,2012.
【13】MIRIGUL A. Reducing shrinkage in injection moldings via the taguchi, anova and neural network methods[J]. Materials and Design, 2010, 31(1):599-604.
【14】郭广思.注塑成型技术[M]. 2版.北京:机械工业出版社,2009. GUO G S. Injection molding technology[M]. 2nd ed. Beijing:China Machine Press,2009.
【15】王博.基于CAE的电池盖注塑模具设计及成型工艺优化[J]. 中国塑料,2015, 29(8):106-110. WANG B. Injection mold design of battery cover and optimization of forming process based on CAE[J]. Chnia Plastics, 2015, 29(8):106-110.
【16】刘文卿.实验设计[M].北京:清华大学出版社,2005. LIU W Q.Design of experiments[M].Beijing:Tsinghua University Press,2005.
【17】ERFAN O, BEHZAD S, SEYED M, et al. Warpage and shrinkage optimization of injection-molded plastic spoon parts for biodegradable polymers using Taguchi,ANOVA and artificial neural network methods[J].Journal of Materials Science & Technology,2016, 32(8):710-720.
相关信息