Rapid Prediction of Steviol Glycosides, Chlorogenic Acids and Moisture in Stevia by Models Based on Near Infrared Diffuse Reflection Spectrometry

摘 要
基于近红外漫反射光谱法,结合偏最小二乘法建立了甜叶菊中甜菊糖苷总量(TSG)、瑞鲍迪苷A (RA)、甜菊苷(STV)、绿原酸总量及水分的定量分析模型。选取不同地区的500个甜叶菊样品,以高效液相色谱法(TSG、RA、STV、绿原酸总量)和烘干法(水分)所得数据为参比,结合样品的近红外光谱图,按照以下条件进行建模:1 TSG模型,校正集385,验证集97,光谱预处理采用多元散射校正(MSC)+一阶导数(1st)+Norris derivative滤波平滑(ND),光谱范围4 090.76~7 085.37 cm-1,主因子数8;2 RA模型,校正集381,验证集94,光谱预处理采用MSC+二阶导数(2nd)+ND,光谱范围4 060.38~6 221.23 cm-1、6 769.51~7 401.24 cm-1,主因子数7;3 STV模型,校正集386,验证集96,光谱预处理采用MSC+1st+ND,光谱范围4 017.86~4 224.39 cm-1、4 370.17~5 172.15 cm-1、5 414.95~9 106.22 cm-1,主因子数5;4绿原酸总量模型,校正集376,验证集95,光谱预处理采用标准正态变量变换(SNV)+1st+Savitzky-Golay卷积平滑(SG),光谱范围4 000.21~5 300.00 cm-1、5 624.00~6 246.90 cm-1、8 746.50~9 373.80 cm-1,主因子数12;5水分模型,校正集389,验证集96,光谱预处理采用MSC+1st+ND,光谱范围4 072.53~7 553.09 cm-1,主因子数8。结果显示:TSG、RA、STV、绿原酸总量和水分模型的校正相关系数、预测相关系数、交叉验证相关系数均大于0.800 0,校正均方根误差、预测均方根误差、交叉验证均方根误差均小于0.500;对各模型进行外部验证,TSG、RA、STV、绿原酸总量和水分的预测值与实测值的拟合相关系数均大于0.880 0;利用模型对甜叶菊样品中TSG、RA、STV、绿原酸总量和水分进行分析,日内精密度(n=6)为0.54%~2.7%,日间精密度(n=6)为1.1%~4.7%。模型用于某试验基地不同生长批次甜叶菊中TSG、RA、绿原酸总量的测定,TSG质量分数为10.40%~13.32%,RA质量分数为4.99%~7.61%,绿原酸质量分数为2.73%~4.07%,测定值的相对标准偏差(n=12)均小于7.0%。










Abstract
Based on near infrared diffuse reflection spectrometry, the models of total steviol glucosides (TSG), rebaudioside A (RA), stevioside (STV), total chlorogenic acids and moisture in stevia combined with partial least square (PLS) method were established. Stevia samples of 500 from different regions were selected, and the data obtained by high performance liquid chromatography (TSG, RA, STV, total chlorogenic acids) and oven drying method (moisture) were used as reference. The models were established combined with near infrared spectra of samples according to the following conditions: 1 TSG model, calibration set of 385, verification set of 97, spectral pretreatments of MSC+1st+ND, spectral range of 4 090.76-7 085.37 cm-1, and principal factor number of 8; 2 RA model, calibration set of 381, verification set of 94, spectral pretreatments of MSC+2nd+ND, spectral ranges of 4 060.38-6 221.23 cm-1, 6 769.51-7 401.24 cm-1, and principal factor number of 7; 3 STV model, calibration set of 386, verification set of 96, spectral pretreatments of MSC+1st+ND, spectral ranges of 4 017.86-4 224.39 cm-1, 4 370.17-5 172.15 cm-1, 5 414.95-9 106.22 cm-1, and principal factor number of 5; 4 total chlorogenic acids model, calibration set of 376, verification set of 95, spectral pretreatments of SNV+1st+SG, spectral ranges of 4 000.21-5 300.00 cm-1, 5 624.00-6 246.90 cm-1, 8 746.50-9 373.80 cm-1, and principal factor number of 12; 5 moisture model, calibration set of 389, verification set of 96, spectral pretreatments of MSC+1st+ND, spectral ranges of 4 072.53-7 553.09 cm-1, and principal factor number of 8. It was shown that the correlation coefficients of correction, prediction and cross validation for TSG, RA, STV, total chlorogenic acids and moisture models were all greater than 0.800 0. The root mean square errors of correction, prediction and cross validation were less than 0.500. External validation of the models showed that the correlation coefficients between predicted values and determined values for TSG, RA, STV, total chlorogenic acids and moisture were greater than 0.880 0. TSG, RA, STV, total chlorogenic acids and moisture in stevia samples were analyzed by their models, and the intra-day precision (n=6) and inter-day precision (n=6) were in the ranges of 0.54%-2.7% and 1.1%-4.7%, respectively. The models have been applied to the determination of TSG, RA and total chlorogenic acids in stevias from different growth batches at a test base, and the mass fractions of TSG, RA and total chlorogenic acids were in the ranges of 10.40%-13.32%, 4.99%-7.61% and 2.73%-4.07%, respectively, with RSDs (n=12) of the determined values were less than 7.0%.
中图分类号 O657.33 DOI 10.11973/lhjy-hx202204015
所属栏目 工作简报
基金项目
收稿日期 2020/8/14
修改稿日期
网络出版日期

作者单位点击查看


备注张苹苹,硕士,研究方向为食品分析与检测
引用该论文: ZHANG Pingping,SHI Wenjie,YANG Qingshan,WANG Lixun,CHENG Yuanxin. Rapid Prediction of Steviol Glycosides, Chlorogenic Acids and Moisture in Stevia by Models Based on Near Infrared Diffuse Reflection Spectrometry[J]. Physical Testing and Chemical Analysis part B:Chemical Analysis, 2022, 58(4): 458~464
张苹苹,石文杰,杨清山,王立勋,程远欣. 基于近红外漫反射光谱法所建的模型快速预测甜叶菊中甜菊糖苷、绿原酸及水分的含量[J]. 理化检验-化学分册, 2022, 58(4): 458~464
共有人对该论文发表了看法,其中:
人认为该论文很差
人认为该论文较差
人认为该论文一般
人认为该论文较好
人认为该论文很好
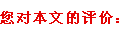





参考文献
【1】杨洋,李启明,高航,等.甜菊糖苷功能特性与应用现状[J].食品工业, 2018,39(11):270-272.
【2】刘琼,潘芸芸,吴卫.甜叶菊化学成分及药理活性研究进展[J].天然产物研究与开发, 2018,30(6):1085-1091.
【3】陈育如,杨凤平,杨帆,等.甜叶菊及甜菊糖的多效功能与保健应用[J].南京师大学报(自然科学版), 2016,39(2):56-60.
【4】PACIFICO S, PICCOLELLA S, NOCERA P, et al. New insights into phenol and polyphenol composition of Stevia rebaudianaleaves[J]. Journal of Pharmaceutical and Biomedical Analysis, 2019,163:45-57.
【5】TYPEK R, DAWIDOWICZ A L, BERNACIK K. Aqueous and alcoholic adducts of steviol and steviol glycosides in food products containing stevia[J]. Food Chemistry, 2020,317:126359.
【6】彭涛,丁辉,宋巧.浅谈甜叶菊的功能及应用前景[J].甘肃农业, 2017(22):37-38.
【7】席利莎,木泰华,孙红男.绿原酸类物质的国内外研究进展[J].核农学报, 2014,28(2):292-301.
【8】杨剀舟.绿原酸抗氧化活性及其自协同抗氧化机理研究[D].北京:中国农业大学, 2016.
【9】李洪辛.绿原酸对小鼠变应性鼻炎的作用及其机制研究[D].长春:吉林大学, 2016.
【10】孙蕊,贾鹏禹,冯胜杰,等.高效液相色谱法测定甜叶菊叶片中甜菊糖苷含量[J].中国食品添加剂, 2019,30(2):131-136.
【11】郭志龙.不同品系甜叶菊中甜菊糖苷和绿原酸类物质含量测定与分析[D].银川:宁夏大学, 2019.
【12】张民达,刘梦婷,李小莹,等.一测多评法测定甜叶菊中6种绿原酸类成分的含量[J].中国现代中药, 2020,22(6):879-883.
【13】刘景彬,刘安军,赵永良,等.甜菊叶中甜菊糖苷检测方法的建立[J].食品科技, 2014,39(10):311-315.
【14】朱吟吟,周凌.高效液相色谱法测定甜叶菊中的3个甜菊醇糖苷的含量[J].药物分析杂志, 2014,34(1):184-189.
【15】李华丽,袁君,郁荣华,等.HPLC法测定甜叶菊中6个酚酸类成分含量[J].药物分析杂志, 2017,37(2):219-223.
【16】荣传兰,孟勇,刘丹.HPLC法同时测定多花蒿中绿原酸类成分的含量[J].中医药导报, 2018,24(8):68-71.
【17】王秋云,朱建猛,胡胜祥,等.近红外在酱香白酒酒醅检测中的应用[J].酿酒科技, 2019(10):91-93.
【18】范林宏,范文翔,韦志强,等.近红外光谱技术结合化学计量学在中药分析中的应用现状[J].中国实验方剂学杂志, 2019,25(24):205-210.
【19】俞延义.近红外光谱技术在地质矿物识别中应用研究[J].中国金属通报, 2019(8):228-228.
【20】李冠.甜叶菊营养品质性状的近红外定标模型的构建及资源筛选[D].杭州:浙江大学, 2012.
【21】荣正勤.甜叶菊糖甙含量近红外光谱定量预测模型的创建及应用[D].杭州:浙江大学, 2011.
【22】汤其坤.重离子辐照甜叶菊抗寒品系筛选及糖苷快速检测方法建立[D].合肥:安徽大学, 2014.
【23】汤其坤,王钰,吴跃进,等.近红外光谱法直接检测甜叶菊叶片甜菊糖苷模型建立[J].光谱学与光谱分析, 2014,34(10):2719-2722.
【2】刘琼,潘芸芸,吴卫.甜叶菊化学成分及药理活性研究进展[J].天然产物研究与开发, 2018,30(6):1085-1091.
【3】陈育如,杨凤平,杨帆,等.甜叶菊及甜菊糖的多效功能与保健应用[J].南京师大学报(自然科学版), 2016,39(2):56-60.
【4】PACIFICO S, PICCOLELLA S, NOCERA P, et al. New insights into phenol and polyphenol composition of Stevia rebaudianaleaves[J]. Journal of Pharmaceutical and Biomedical Analysis, 2019,163:45-57.
【5】TYPEK R, DAWIDOWICZ A L, BERNACIK K. Aqueous and alcoholic adducts of steviol and steviol glycosides in food products containing stevia[J]. Food Chemistry, 2020,317:126359.
【6】彭涛,丁辉,宋巧.浅谈甜叶菊的功能及应用前景[J].甘肃农业, 2017(22):37-38.
【7】席利莎,木泰华,孙红男.绿原酸类物质的国内外研究进展[J].核农学报, 2014,28(2):292-301.
【8】杨剀舟.绿原酸抗氧化活性及其自协同抗氧化机理研究[D].北京:中国农业大学, 2016.
【9】李洪辛.绿原酸对小鼠变应性鼻炎的作用及其机制研究[D].长春:吉林大学, 2016.
【10】孙蕊,贾鹏禹,冯胜杰,等.高效液相色谱法测定甜叶菊叶片中甜菊糖苷含量[J].中国食品添加剂, 2019,30(2):131-136.
【11】郭志龙.不同品系甜叶菊中甜菊糖苷和绿原酸类物质含量测定与分析[D].银川:宁夏大学, 2019.
【12】张民达,刘梦婷,李小莹,等.一测多评法测定甜叶菊中6种绿原酸类成分的含量[J].中国现代中药, 2020,22(6):879-883.
【13】刘景彬,刘安军,赵永良,等.甜菊叶中甜菊糖苷检测方法的建立[J].食品科技, 2014,39(10):311-315.
【14】朱吟吟,周凌.高效液相色谱法测定甜叶菊中的3个甜菊醇糖苷的含量[J].药物分析杂志, 2014,34(1):184-189.
【15】李华丽,袁君,郁荣华,等.HPLC法测定甜叶菊中6个酚酸类成分含量[J].药物分析杂志, 2017,37(2):219-223.
【16】荣传兰,孟勇,刘丹.HPLC法同时测定多花蒿中绿原酸类成分的含量[J].中医药导报, 2018,24(8):68-71.
【17】王秋云,朱建猛,胡胜祥,等.近红外在酱香白酒酒醅检测中的应用[J].酿酒科技, 2019(10):91-93.
【18】范林宏,范文翔,韦志强,等.近红外光谱技术结合化学计量学在中药分析中的应用现状[J].中国实验方剂学杂志, 2019,25(24):205-210.
【19】俞延义.近红外光谱技术在地质矿物识别中应用研究[J].中国金属通报, 2019(8):228-228.
【20】李冠.甜叶菊营养品质性状的近红外定标模型的构建及资源筛选[D].杭州:浙江大学, 2012.
【21】荣正勤.甜叶菊糖甙含量近红外光谱定量预测模型的创建及应用[D].杭州:浙江大学, 2011.
【22】汤其坤.重离子辐照甜叶菊抗寒品系筛选及糖苷快速检测方法建立[D].合肥:安徽大学, 2014.
【23】汤其坤,王钰,吴跃进,等.近红外光谱法直接检测甜叶菊叶片甜菊糖苷模型建立[J].光谱学与光谱分析, 2014,34(10):2719-2722.
相关信息