A deep learning model based on multi-source feature fusion and defect type recognition method for complex components

摘 要
复杂构件内部缺陷的类型识别对于保证装备制造质量及安全可靠运行具有重要的意义。针对现有深度学习模型用于缺陷类型识别时存在局部特征提取较差、缺乏考虑缺陷经验特性以及特征信息丢失的问题,提出了一种融合先验特征、全局特征以及ReliefF-Pooling策略的缺陷类型识别方法;实现了缺陷几何、纹理等先验特征与卷积神经网络全局特征的融合分析,并通过构建基于ReliefF-Pooling的特征优化方法,实现不同权重特征信息优化利用;最后,以某航天企业实际的复杂构件内部缺陷的射线检测为例进行了验证。试验结果表明,所提方法可以有效提升复杂构件内部缺陷的类型识别精度。








Abstract
The internal defect type recognition of complex components is of great significance to ensure equipment manufacturing quality and safe and reliable operation. Aiming at the problems of poor local feature extraction, lack of considering the empirical characteristics of defects and loss of feature information in the existing deep learning model for defect type recognition, a defect type recognition method based on prior features, global features and ReliefF-Pooling strategy is proposed. The fusion analysis of prior features such as defect geometry, texture and global features of convolutional neural network (CNN) is realized, and the feature optimization method based on ReliefF-Pooling is constructed to optimize the utilization of feature information with different weights. Finally, as an example, the actual radiographic testing of internal defects in complex components in an aerospace enterprise is used to verify the proposed method. The experimental results show that the proposed method can effectively improve the type recognition accuracy of internal defects in complex components.
中图分类号 TP391 TG115.28 DOI 10.11973/wsjc202302003
所属栏目 试验研究
基金项目
收稿日期 2022/8/5
修改稿日期
网络出版日期

作者单位点击查看

联系人作者姜洪权(jhqxjtu@163.com)

备注程虎跃(1998-),男,博士研究生,主要研究方向为机器视觉与智能制造、无损检测与缺陷识别技术
引用该论文: CHENG Huyue,LIU Zhen,SHI Zhiguang,WANG Yonghong,JIANG Hongquan,YANG Deyan,GAO Jianmin,ZHI Zelin. A deep learning model based on multi-source feature fusion and defect type recognition method for complex components[J]. Nondestructive Testing, 2023, 45(2): 12~17
程虎跃,刘贞,史志光,王永红,姜洪权,杨得焱,高建民,支泽林. 一种多源特征融合深度学习模型及复杂构件[J]. 无损检测, 2023, 45(2): 12~17
共有人对该论文发表了看法,其中:
人认为该论文很差
人认为该论文较差
人认为该论文一般
人认为该论文较好
人认为该论文很好
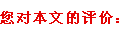





参考文献
【1】朱镇, 陈晓娟, 王兆云.核电站用高磅级阀体铸件的全体积射线检测[J].无损检测, 2022, 44(1):49-52, 59.
【2】蔡彪, 沈宽, 付金磊, 等.基于Mask R-CNN的铸件X射线DR图像缺陷检测研究[J].仪器仪表学报, 2020, 41(3):61-69.
【3】JIANG H Q, WANG R X, GAO Z Y, et al.Classification of weld defects based on the analytical hierarchy process and Dempster-Shafer evidence theory[J].Journal of Intelligent Manufacturing, 2019, 30(4):2013-2024.
【4】DA SILVA R R, MERY D.The state of the art of weld seam radiographic testing:part II, pattern recognition[J].Materials Evaluation, 2007, 65(8):833-838.
【5】JIANG H Q, ZHAO Y L, GAO J M, et al.Weld defect classification based on texture features and principal component analysis[J].Insight, 2016, 58:194-200.
【6】MEKHALFA F, NACEREDDINE N.Multiclass classification of weld defects in radiographic images based on support vector machines[C]//2014 Tenth International Conference on Signal-Image Technology and Internet-Based Systems.Marrakech, Morocco:IEEE, 2015:1-6.
【7】YANG L, JIANG H Q.Weld defect classification in radiographic images using unified deep neural network with multi-level features[J].Journal of Intelligent Manufacturing, 2021, 32(2):459-469.
【8】姜洪权, 贺帅, 高建民, 等.一种改进卷积神经网络模型的焊缝缺陷识别方法[J].机械工程学报, 2020, 56(8):235-242.
【9】雷铮强, 王维斌, 李立军.中俄东线管道射线检测底片图像的智能识别[J].无损检测, 2022, 44(4):73-78.
【10】LU Q Y, WONG C H.Additive manufacturing process monitoring and control by non-destructive testing techniques:challenges and in-process monitoring[J].Virtual and Physical Prototyping, 2018, 13(2):39-48.
【11】AOUAT S, AIT-HAMMI I, HAMOUCHENE I.A new approach for texture segmentation based on the Gray Level Co-occurrence Matrix[J].Multimedia Tools and Applications, 2021, 80(16):24027-24052.
【12】CARLOS E, BERTHA G, AMPARO A, et al.Scalable feature selection using ReliefF aided by locality-sensitive hashing[J].International Journal of Intelligent Systems, 2021, 36(11):6161-6179.
【2】蔡彪, 沈宽, 付金磊, 等.基于Mask R-CNN的铸件X射线DR图像缺陷检测研究[J].仪器仪表学报, 2020, 41(3):61-69.
【3】JIANG H Q, WANG R X, GAO Z Y, et al.Classification of weld defects based on the analytical hierarchy process and Dempster-Shafer evidence theory[J].Journal of Intelligent Manufacturing, 2019, 30(4):2013-2024.
【4】DA SILVA R R, MERY D.The state of the art of weld seam radiographic testing:part II, pattern recognition[J].Materials Evaluation, 2007, 65(8):833-838.
【5】JIANG H Q, ZHAO Y L, GAO J M, et al.Weld defect classification based on texture features and principal component analysis[J].Insight, 2016, 58:194-200.
【6】MEKHALFA F, NACEREDDINE N.Multiclass classification of weld defects in radiographic images based on support vector machines[C]//2014 Tenth International Conference on Signal-Image Technology and Internet-Based Systems.Marrakech, Morocco:IEEE, 2015:1-6.
【7】YANG L, JIANG H Q.Weld defect classification in radiographic images using unified deep neural network with multi-level features[J].Journal of Intelligent Manufacturing, 2021, 32(2):459-469.
【8】姜洪权, 贺帅, 高建民, 等.一种改进卷积神经网络模型的焊缝缺陷识别方法[J].机械工程学报, 2020, 56(8):235-242.
【9】雷铮强, 王维斌, 李立军.中俄东线管道射线检测底片图像的智能识别[J].无损检测, 2022, 44(4):73-78.
【10】LU Q Y, WONG C H.Additive manufacturing process monitoring and control by non-destructive testing techniques:challenges and in-process monitoring[J].Virtual and Physical Prototyping, 2018, 13(2):39-48.
【11】AOUAT S, AIT-HAMMI I, HAMOUCHENE I.A new approach for texture segmentation based on the Gray Level Co-occurrence Matrix[J].Multimedia Tools and Applications, 2021, 80(16):24027-24052.
【12】CARLOS E, BERTHA G, AMPARO A, et al.Scalable feature selection using ReliefF aided by locality-sensitive hashing[J].International Journal of Intelligent Systems, 2021, 36(11):6161-6179.
相关信息